The evolution of Generative AI (GenAI) has transformed language translation, offering promising solutions for bridging linguistic gaps between complex languages like Japanese and Chinese. While GenAI tools are redefining the translation landscape, their capabilities vary widely depending on context, cultural nuances, and the intricacies of each language. This article takes an in-depth look at what works, what doesn’t, and what additional steps can further enhance translation quality.
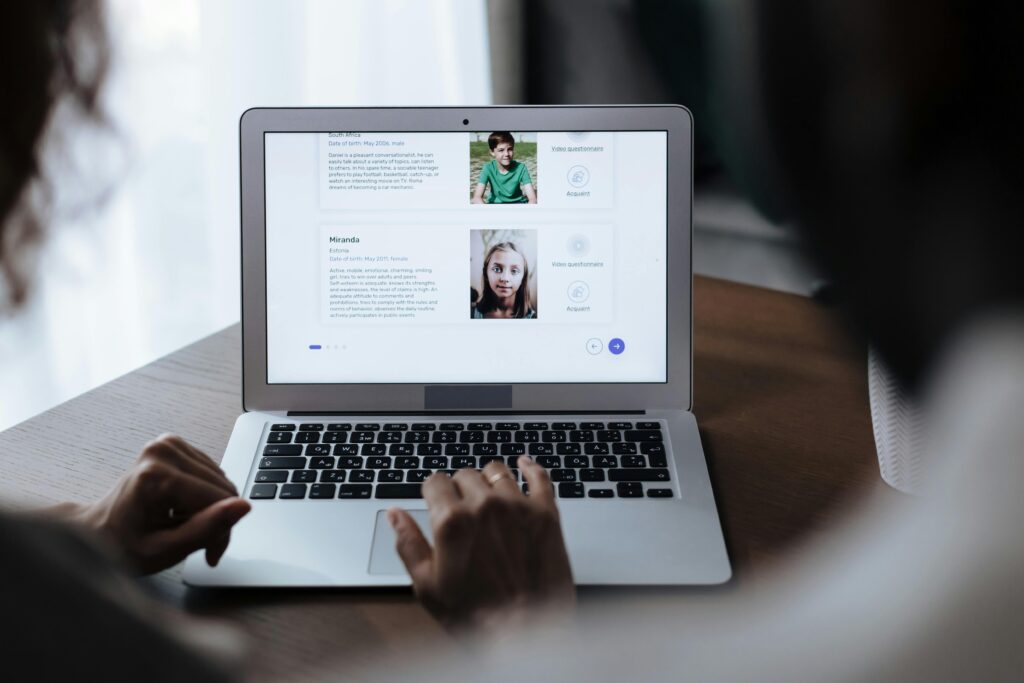
Understanding the Linguistic Complexities
Divergent Linguistic Structures:
Japanese and Chinese represent two distinct language families with different grammatical structures, syntax, and writing systems. Japanese utilizes a combination of kanji (borrowed Chinese characters), hiragana, and katakana, while Chinese relies solely on logographic characters. This intrinsic difference means that translating between the two isn’t just about replacing words—it’s about conveying meaning, tone, and context accurately.
Idiomatic Expressions and Cultural Context:
Both languages are rich in idioms and culturally loaded expressions. For example, a direct translation might miss the underlying sentiment or social nuance. The challenge is ensuring that translated phrases resonate with the intended cultural meaning, rather than being a literal, and often confusing, word-for-word substitute.
How GenAI is Revolutionizing Translation
Advances in Neural Machine Translation:
Recent breakthroughs in GenAI leverage deep learning and neural networks, enabling systems to learn from vast datasets of bilingual text. These models have significantly improved the fluency and contextual accuracy of translations by:
- Contextual Embeddings: Capturing the surrounding context of words to choose the most relevant meaning.
- Sequence-to-Sequence Models: Enhancing the ability to translate long sentences while preserving grammatical structure.
- Continuous Learning: Allowing the models to adapt to new linguistic trends and evolving language use over time.
Custom Training for Domain-Specific Translation:
One of the most effective approaches involves fine-tuning GenAI models with domain-specific datasets. For instance, training on legal, technical, or literary texts in Japanese and Chinese helps the system understand the context-specific terminology and style, thereby increasing accuracy.
What Works: Strengths of GenAI in Japanese-Chinese Translation
Speed and Efficiency:
GenAI can translate large volumes of text quickly, making it a valuable tool for initial drafts or content that requires rapid turnaround. This efficiency is particularly beneficial in contexts like social media, customer support, or live events where timeliness is crucial.
Improved Contextual Understanding:
Modern GenAI models are better at interpreting the broader context of sentences, which helps in selecting the right words and phrases that fit naturally into the target language. This capability is especially important when dealing with languages that rely heavily on context, like Japanese and Chinese.
Scalability and Continuous Improvement:
As more bilingual data becomes available, GenAI systems improve over time. Continuous training allows these models to adapt to changes in language use and to learn from real-world applications. Over time, this leads to more refined translations that increasingly capture subtle nuances.
Hybrid Translation Workflows:
Many successful implementations now combine GenAI with human oversight. Machine-generated translations can serve as a first draft, which human experts then refine for accuracy and cultural relevance. This collaborative approach harnesses the speed of AI while ensuring high-quality, context-aware outcomes.
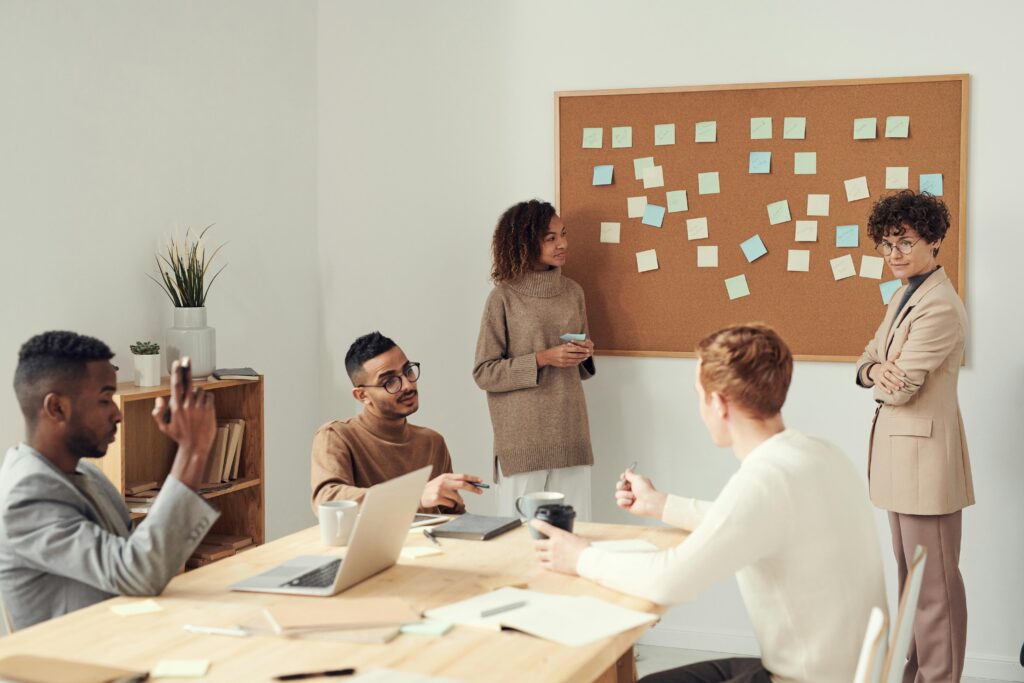
What Doesn’t Work: Limitations and Challenges
Struggling with Nuances and Ambiguities:
Despite advances, GenAI still grapples with the nuanced meanings behind idiomatic expressions and culturally loaded phrases. A model might translate a Japanese proverb literally into Chinese, losing the metaphorical significance or failing to convey the intended emotion.
Handling Contextual Shifts:
Language is fluid, and meanings can shift depending on context. GenAI might excel in translating static texts but encounter difficulties when dealing with dynamic content where the context rapidly changes, such as in live conversations or creative literature.
Bias and Inconsistency:
GenAI models learn from the data they are trained on. If the training datasets contain biases or are unbalanced in terms of language style, the output may inherit these issues. Inconsistent translation styles or unexpected word choices can lead to mistranslations, especially in formal or technical documents.
Lack of Deep Cultural Insight:
While AI can process patterns and context, it often lacks the intuitive understanding of cultural subtleties that a native speaker or expert translator brings. This shortfall can result in translations that, while grammatically correct, might feel off or fail to resonate with the target audience.
Enhancing Translation Quality: Best Practices and Emerging Solutions
Integrating Cultural Intelligence:
Future GenAI systems could incorporate cultural intelligence modules that explicitly handle regional idioms, historical contexts, and local expressions. Collaborative efforts between linguists and AI developers can help integrate cultural databases into the models, leading to more contextually aware translations.
Leveraging User Feedback Loops:
Implementing robust feedback mechanisms where native speakers can review and correct translations can drive iterative improvements. This crowdsourced model can help identify recurring errors and train AI systems to avoid similar mistakes in the future.
Domain-Specific Customization:
Organizations can further improve accuracy by customizing GenAI models for specific sectors such as finance, healthcare, or literature. Tailoring the training data to the context ensures that the translation captures both the technical language and the stylistic nuances needed in each domain.
Enhanced Post-Editing Processes:
While GenAI provides a solid baseline, integrating comprehensive human post-editing remains crucial. Expert translators can fine-tune the output, ensuring that the final product meets the high standards required for professional or sensitive communications.
Future Directions in GenAI Translation
Multimodal Translation Systems:
Emerging research is exploring the integration of multimodal data—combining text, audio, and even visual cues—to improve translation accuracy further. Such systems could provide contextual hints from accompanying images or tone of voice, enhancing understanding in real-time conversations.
Adaptive Learning Models:
Future GenAI models may incorporate adaptive learning techniques that adjust in real time based on immediate feedback. This could be especially useful in interactive settings like live translations or collaborative projects, where the system continuously refines its output based on user interactions.
Interdisciplinary Collaboration:
The next wave of innovation will likely stem from closer collaboration between AI experts, linguists, and cultural scholars. Combining technical expertise with deep cultural understanding promises to bridge the remaining gaps in machine translation, paving the way for systems that not only translate but also interpret meaning accurately.
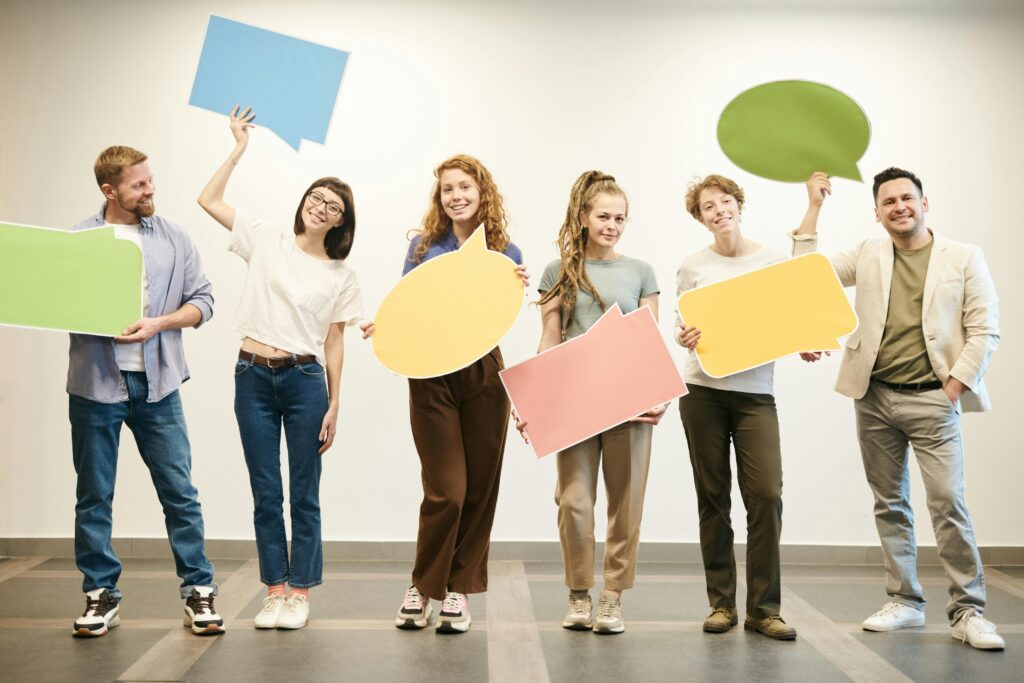
Frequently Asked Questions (FAQs)
Q1: What are the key challenges in translating between Japanese and Chinese using GenAI?
A: The main challenges include managing the structural differences between the languages, accurately capturing idiomatic expressions, and conveying the cultural context behind words and phrases.
Q2: How does GenAI improve translation quality compared to traditional tools?
A: GenAI leverages deep learning and contextual embeddings to understand the broader context of a sentence, offering more fluid and context-aware translations. Continuous training on large datasets also helps improve its accuracy over time.
Q3: Can GenAI handle domain-specific language effectively?
A: Yes, when GenAI models are fine-tuned with domain-specific data, they perform significantly better in translating specialized content such as technical documents, legal texts, or medical literature.
Q4: What are the limitations of current GenAI translation models?
A: Current limitations include struggles with cultural nuances, ambiguity in idiomatic expressions, bias from training data, and occasional inconsistency in output, which necessitates human post-editing for high-stakes translations.
Q5: How can translation quality be further enhanced?
A: Enhancing quality involves integrating cultural intelligence, implementing robust feedback loops, leveraging domain-specific customization, and maintaining comprehensive human post-editing processes.
Q6: What future developments can we expect in GenAI translation?
A: Future advancements may include multimodal translation systems that use audio and visual cues, adaptive learning models that refine output in real time, and deeper interdisciplinary collaborations to merge linguistic expertise with AI innovation.
By harnessing the power of GenAI for Japanese-Chinese translation, we are witnessing both impressive strides and clear challenges. While current systems offer speed and contextual awareness, ongoing improvements and strategic human intervention remain critical to achieving translations that are both accurate and culturally resonant. This balanced approach promises a future where machine translation not only bridges language gaps but also brings people closer together through deeper mutual understanding.
Sources Toward Data Science