In today’s interconnected world, language should no longer be a barrier to communication and knowledge-sharing. Thanks to advancements in Artificial Intelligence (AI), Natural Language Processing (NLP) has transformed the way we summarize and translate text across multiple languages. AI-driven multilingual text summarization and translation are now making information more accessible than ever, breaking down linguistic walls and enabling seamless global interaction.
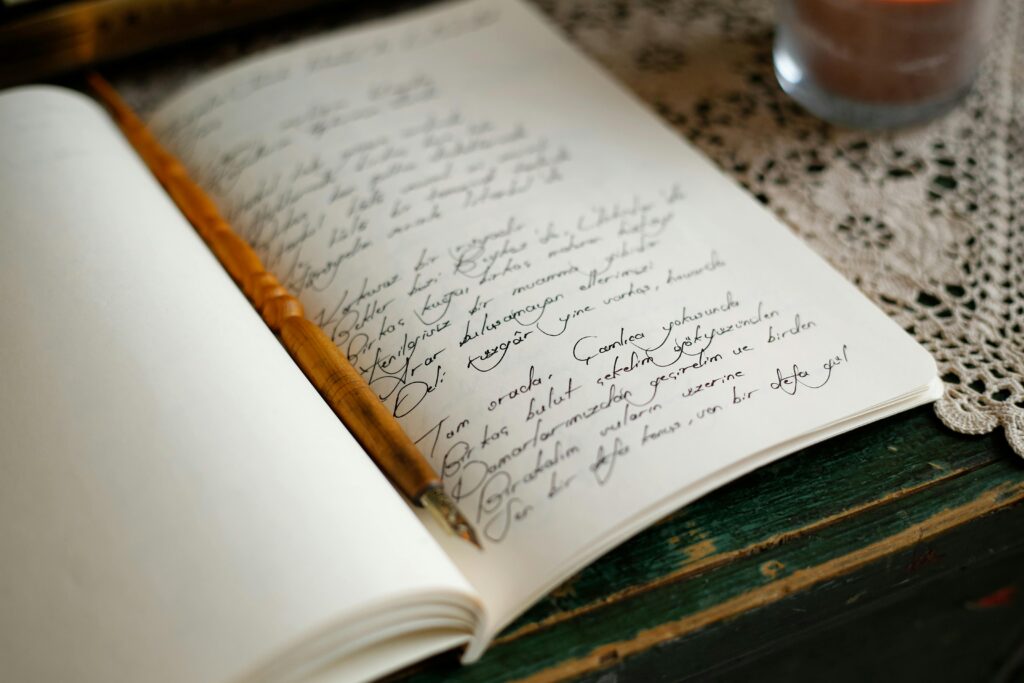
What is Multilingual Text Summarization and Translation?
Multilingual text summarization refers to the AI-powered process of condensing lengthy texts into concise summaries, either in the same language (monolingual summarization) or in a different language (cross-lingual summarization). AI models can analyze large volumes of text and extract key insights, saving users time while ensuring they grasp the essential information.
On the other hand, AI-driven translation converts text from one language to another while preserving meaning, tone, and context. Traditional machine translation systems often struggled with accuracy and fluency. However, modern AI models—such as Google’s Neural Machine Translation (GNMT) and OpenAI’s GPT models—have significantly improved translation quality by considering entire sentences rather than individual words.
How AI is Transforming Multilingual Summarization and Translation
1. The Rise of Transformer-Based AI Models
One of the biggest breakthroughs in NLP is the transformer architecture, introduced in 2017. Transformers process words in relation to all other words in a sentence, rather than analyzing them one by one. This enables AI to better understand context and generate more accurate summaries and translations. Popular AI models like BERT, GPT, and T5 are built on this foundation.
2. Real-Time and Context-Aware Translation
Companies like Meta and Google have been developing AI models that provide real-time translations with emotion and tone preservation. Meta’s SEAMLESSM4T, for example, allows voice translation in over 100 languages while keeping the speaker’s original emotions intact.
3. AI-Powered Automatic Summarization
AI models can now summarize large volumes of text within seconds, making them useful for professionals, researchers, and businesses that need quick insights. These models can process news articles, legal documents, and research papers, producing high-quality summaries in multiple languages.
4. Overcoming Low-Resource Language Challenges
While AI performs well with major languages like English, Spanish, and Chinese, low-resource languages (languages with limited digital text data) still pose a challenge. Efforts are being made to improve AI proficiency in these languages through data collection, model fine-tuning, and synthetic text generation.
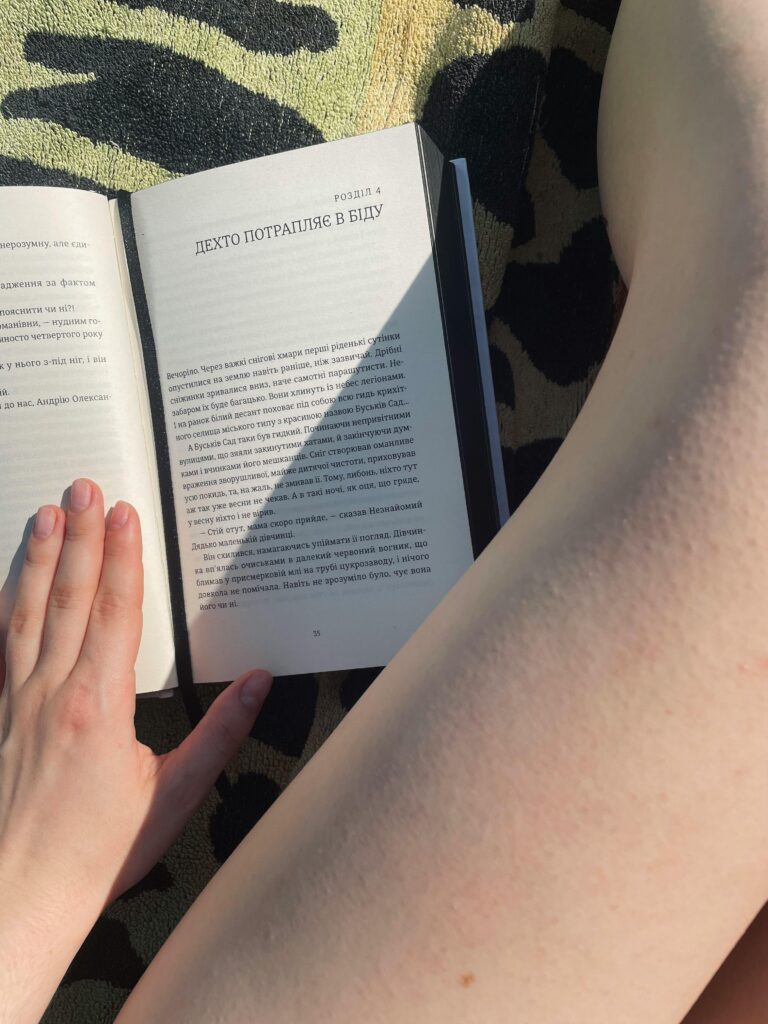
Challenges and Ethical Considerations
Despite these advancements, AI-based translation and summarization still have limitations:
- Cultural and Contextual Understanding: AI can struggle with idioms, slang, and culturally specific expressions, which may lead to inaccurate or awkward translations.
- Bias in AI Models: AI models are trained on existing data, which can introduce biases in translations and summaries. Researchers are actively working on making AI more fair and unbiased.
- Security and Privacy Risks: Since AI translation tools process large amounts of text, data privacy remains a concern, especially in sensitive fields like law and healthcare.
The Future of AI in Language Processing
The future of AI-driven NLP looks promising, with researchers focusing on multimodal AI—models that can process text, speech, and images simultaneously. These advancements will allow AI to better understand context, emotions, and user intent, making translations and summaries even more accurate.
Additionally, AI’s growing ability to generate human-like content will further enhance machine translation, making it almost indistinguishable from human-generated text.
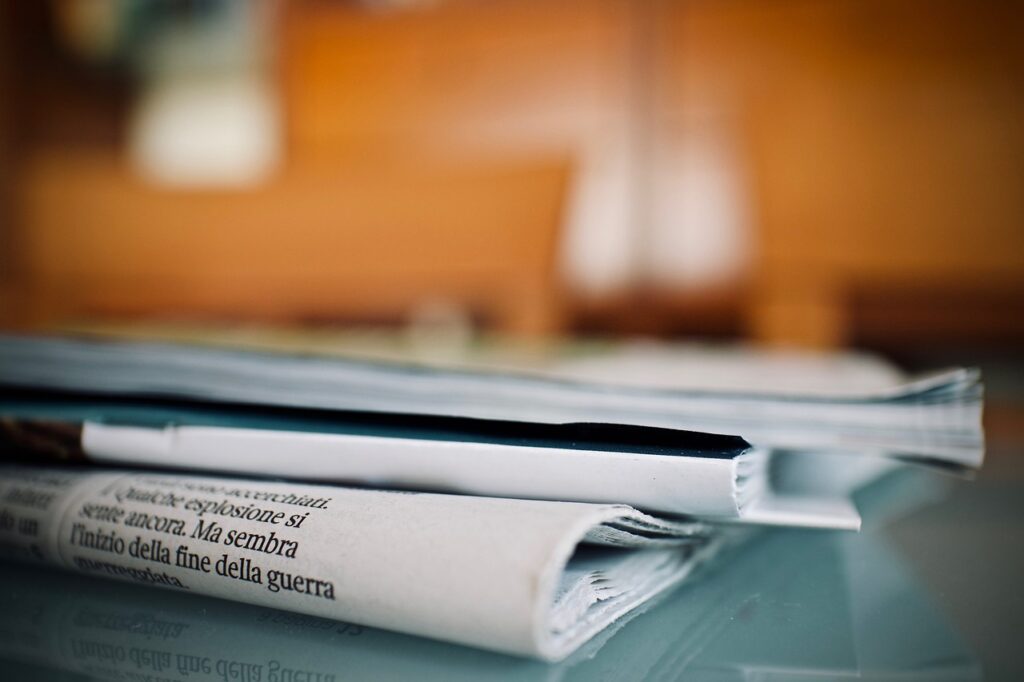
Frequently Asked Questions (FAQ)
1. How does AI improve multilingual text summarization?
AI models analyze large amounts of text, extract key points, and generate concise summaries while maintaining the core message and context.
2. What makes AI translations better than traditional methods?
AI-powered translations use deep learning and neural networks to process sentences holistically rather than translating word-by-word, making translations more fluent and natural.
3. Can AI handle complex languages and dialects?
Yes, but with limitations. AI performs best with languages that have abundant digital data, while rare languages and dialects still require more training data and model improvements.
4. Will AI replace human translators?
Not entirely. While AI is great for general translations, human translators are still needed for literary works, legal documents, and other cases where deep cultural and contextual understanding is crucial.
5. How is AI ensuring fairness in translations?
Researchers are developing bias-detection algorithms and fine-tuning AI models to reduce gender, cultural, and racial biases in translations.
Conclusion
AI-driven multilingual summarization and translation are shaping a future where language is no longer a barrier. While challenges remain, advancements in transformer-based AI models, real-time translation, and low-resource language processing are revolutionizing the way we communicate across cultures.
As AI continues to evolve, we can expect even more accurate, fluent, and context-aware translations—bringing the world closer together through the power of language. 🌍✨
Sources CEOWorld